When AI Meets Quantum: The Rise of Probabilistic Supremacy
- Sandeep Deb Varman
- Mar 7
- 2 min read
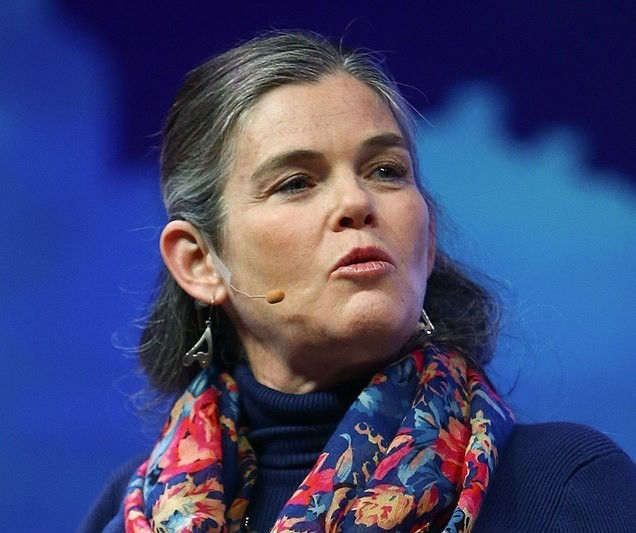
Daphne Koller's breakthrough in probabilistic learning laid groundwork that now stands at a fascinating crossroads. As quantum computing emerges from theoretical physics into engineering reality, it presents an intriguing proposition: What happens when AI's pattern recognition meets quantum mechanical superposition?
The computational demands of contemporary AI systems are staggering. A single training run of an advanced language model consumes enough energy to power a small city for a month. Yet quantum computing suggests a paradigm where this computational ceiling shatters, replacing brute-force calculation with quantum probability waves.
Consider how quantum mechanics fundamentally differs from classical computation. Where classical bits must choose between 0 and 1, quantum bits exist in probabilistic superpositions. This isn't merely a technical distinction—it represents a fundamental shift in how computation interfaces with physical reality.
Early quantum-AI hybrid systems are already demonstrating provocative results in optimization problems. These systems don't just process information faster; they navigate probability spaces that classical computers cannot meaningfully access. The implications for machine learning are profound: training algorithms that don't just learn from data, but exist in a superposition of all possible learning states. (yes, we're struggling to wrap our heads around it too)
The technical challenges remain formidable. Quantum decoherence—the tendency of quantum systems to lose their quantum properties through environmental interaction—presents a fundamental engineering challenge. Error correction requires an elegant dance between classical and quantum systems, each compensating for the other's limitations.
Yet the potential applications verge on the revolutionary. Imagine molecular modeling systems that don't just simulate drug interactions but explore the quantum mechanical properties of biological systems directly. Financial models that don't predict market behavior but exist in a superposition of all possible market states. Climate models that handle atmospheric quantum effects directly rather than through classical approximations.
The timeline to quantum AI supremacy remains uncertain, but the theoretical foundations are solidifying. We're not merely waiting for more powerful computers—we're developing a new computational paradigm where probability isn't just a tool for understanding uncertainty, but the fundamental language of computation itself.
This convergence of quantum computing and AI doesn't just promise faster computers; it suggests a fundamental reimagining of what computation means in a probabilistic universe.
QuLearnLabs is supported by the EIT Deep Tech Talent Initiative of the European Institute of Innovation and Technology (EIT)
Comments